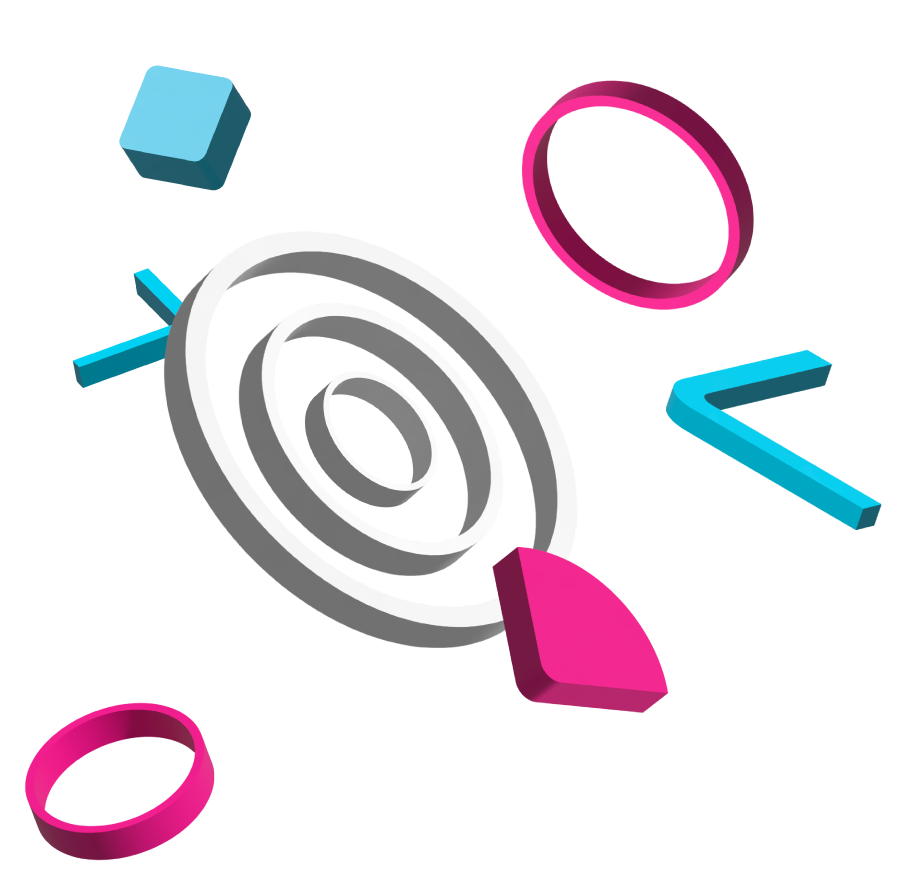
Our Research
With the WestAI Service Center, a consortium of excellent scientific institutions in North Rhine-Westphalia has been formed under the leadership of the University of Bonn. The center brings together complementary expertise from various fields of AI application in order to develop new powerful and efficient multimodal AI models for Germany.
Our research focuses on transfer learning and multimodal AI.
At WestAI, we conduct research in the field of artificial intelligence and machine learning for the application of deep learning methods. Deep learning uses deep neural networks and large amounts of data to solve problems. More specifically, we are looking at the sub-area of transfer learning. This method makes it possible to use existing knowledge from an existing AI solution to solve a new problem more efficiently. Artificial neural networks are used, which saves time and resources.
Our research focusses on the transfer learning of multimodal AI models – these are AI models that can process sensor, audio or video data in addition to text, for example. The aim is to advance the state of the art in multimodal AI by investigating the scalability, efficiency, transferability and continuous improvement of learning algorithms.
Our research framework covers the following areas:
Scalable data management and integration
Research into techniques for automatic data pre-processing and generation, especially for heterogeneous and multimodal data types. The consideration of data protection and data sovereignty in real applications plays a central role here.
Large-scale model training
Developing efficient learning methods for training across multiple compute nodes and exploring different model architectures and model training methods for the next generation of accelerated computing.
Efficient transfer learning
Systematic investigation of the conditions of transfer learning from pre-trained models to different domains or application areas, taking into account factors such as model and data scope, architecture and transfer efficiency.
Model compression for low-resource environments
Investigation of methods for compressing pre-trained and ‘transferred’ models (after transfer learning) while maintaining the performance of a model. Research into the use of models on special hardware for energy-efficient execution is also being realised.
Continuous learning
Investigating the ongoing development of large AI models through continuous learning from transferred and compressed models. The scalability and possible applications in federated learning environments are being researched.
Our publications
PermutoSDF: Fast Multi-View Reconstruction with Implicit Surfaces using Permutohedral Lattices
Radu Alexandru Rosu and Sven Behnke. PermutoSDF: Fast Multi-View Reconstruction with Implicit Surfaces using Permutohedral Lattices. In IEEE/CVF Conference on Computer Vision and Pattern Recognition (CVPR), Vancouver, Canada, June 2023.
Reproducible scaling laws for contrastive language-image learning
Mehdi Cherti, Romain Beaumont, Ross Wightman, Mitchell Wortsman, Gabriel Ilharco, Cade Gordon, Christoph Schuhmann, Ludwig Schmidt, and Jenia Jitsev. Reproducible scaling laws for contrastive language-image learning. Proceedings of the IEEE/CVF Conference on Computer Vision and Pattern Recognition (CVPR), 2023.
External Camera-based Mobile Robot Pose Estimation for Collaborative Perception with Smart Edge Sensors
Simon Bultmann, Raphael Memmesheimer, and Sven Behnke. External Camera-based Mobile Robot Pose Estimation for Collaborative Perception with Smart Edge Sensors in IEEE International Conference on Robotics and Automation (ICRA), London, UK, June 2023.
Unified shape and appearance reconstruction with joint camera parameter refinement
Julian Kaltheuner, Patrick Stotko, and Reinhard Klein. Unified shape and appearance reconstruction with joint camera parameter refinement. Graphical Models, Volume 129, 2023.
Social Diffusion: Long-term Multiple Human Motion Anticipation
Julian Tanke, Linguang Zhang, Amy Zhao, Chengcheng Tang, Yujun Cai, Lezi Wang, Po-Chen Wu, Juergen Gall, and Cem Keskin. Social Diffusion: Long-term Multiple Human Motion Anticipation.
Rank Collapse Causes Over-Smoothing and Over-Correlation in Graph Neural Networks
Andreas Roth and Thomas Liebig. Rank Collapse Causes Over-Smoothing and Over-Correlation in Graph Neural Networks. arXiv preprint arXiv:2308.16800.
Curvature-based Pooling within Graph Neural Networks
Cedric Sanders, Andreas Roth, and Thomas Liebig. Curvature-based Pooling within Graph Neural Networks. arXiv preprint arXiv:2308.16516.
LAION-5B: An open large-scale dataset for training next generation image-text models
Christoph Schuhmann, Romain Beaumont, Richard Vencu, Cade Gordon, Ross Wightman, Mehdi Cherti, Theo Coombes, Aarush Katta, Clayton Mullis, Mitchell Wortsman, Patrick Schramowski, Srivatsa Kundurthy, Katherine Crowson, Ludwig Schmidt, Robert Kaczmarczyk, Jenia Jitsev. LAION-5B: An open large-scale dataset for training next generation image-text models. Advances in Neural Information Processing Systems 35 (NeurIPS 2022), 25278-25294.
DataComp: In search of the next generation of multimodal datasets
Samir Yitzhak Gadre, Gabriel Ilharco, Alex Fang, Jonathan Hayase, Georgios Smyrnis, Thao Nguyen, Ryan Marten, Mitchell Wortsman, Dhruba Ghosh, Jieyu Zhang, Eyal Orgad, Rahim Entezari, Giannis Daras, Sarah Pratt, Vivek Ramanujan, Yonatan Bitton, Kalyani Marathe, Stephen Mussmann, Richard Vencu, Mehdi Cherti, Ranjay Krishna, Pang Wei Koh, Olga Saukh, Alexander Ratner, Shuran Song, Hannaneh Hajishirzi, Ali Farhadi, Romain Beaumont, Sewoong Oh, Alex Dimakis, Jenia Jitsev, Yair Carmon, Vaishaal Shankar, Ludwig Schmidt; DataComp: In search of the next generation of multimodal datasets. NeurIPS 2023.
FPO++: Efficient Encoding and Rendering of Dynamic Neural Radiance Fields by Analyzing and Enhancing Fourier PlenOctrees
Saskia Rabich, Patrick Stotko, and Reinhard Klein, “FPO++: Efficient encoding and rendering of dynamic neural radiance fields by analyzing and enhancing Fourier PlenOctrees,” arXiv (Cornell University), Jan. 2023, doi: 10.48550/arxiv.2310.20710.
On the Stability of Neural Segmentation in Radiology
Moritz Wolter, Lokesh Veeramacheneni, Bettina Baeßler, Ulrike Attenberger and Barbara Wichtmann, ”On the Stability of Neural Segmentation in Radiology”, ESANN, 2024,
Word Sense Disambiguation as a Game of Neurosymbolic Darts
Tiansi Dong and Rafet Sifa, “Word sense disambiguation as a game of neurosymbolic darts,” arXiv (Cornell University), Jan. 2023, doi: 10.48550/arxiv.2307.16663.
Learning subsurface scattering solutions of tightly-packed granular media using optimal transport
Domenic Zingsheim and Reinhard Klein, “Learning subsurface scattering solutions of tightly-packed granular media using optimal transport,” Computers & Graphics, vol. 119, p. 103895, Feb. 2024, doi: 10.1016/j.cag.2024.103895.
Physics-guided Shape-from-Template: Monocular Video Perception through Neural Surrogate Models
David Stotko, Nils Wandel, and Reinhard Klein, “Physics-guided Shape-from-Template: Monocular Video Perception through Neural Surrogate Models,” arXiv (Cornell University), Jan. 2023, doi: 10.48550/arxiv.2311.12796.
TraM-NeRF: Tracing Mirror and Near-Perfect Specular Reflections through Neural Radiance Fields
Van Holland Leif, Ruben Bliersbach, Jan U. Müller, Patrick Stotko, and Reinhard Klein, “TraM-NeRF: Tracing Mirror and Near-Perfect Specular Reflections through Neural Radiance Fields,” arXiv (Cornell University), Jan. 2023, doi: 10.48550/arxiv.2310.10650.
SLCF-Net: Sequential LiDAR-Camera Fusion for Semantic Scene Completion using a 3D Recurrent U-Net
Helin Cao and Sven Behnke, “SLCF-Net: Sequential LiDAR-Camera Fusion for Semantic Scene Completion using a 3D Recurrent U-Net,” arXiv (Cornell University), Mar. 2024, doi: 10.48550/arxiv.2403.08885.
Learning from SAM: Harnessing a Foundation Model for Sim2Real Adaptation by Regularization
Mayara E. Bonani, Max Schwarz, and Sven Behnke, “Learning from SAM: Harnessing a Segmentation Foundation Model for Sim2Real Domain Adaptation through Regularization,” arXiv (Cornell University), Jan. 2023, doi: 10.48550/arxiv.2309.15562.
Post-Processing Independent Evaluation of Sound Event Detection Systems
Janek Ebbers, Reinhold Haeb-Umbach, Paderborn University, Romain Serizel, and Universit´e de Lorraine, CNRS, Inria, Loria, “Post-Processing independent evaluation of sound event detection systems,” Journal-article, 2023. [Online]. https://dcase.community/documents/workshop2023/proceedings/DCASE2023Workshop_Ebbers_62.pdf
pFedV: Mitigating Feature Distribution Skewness via Personalized Federated Learning with Variational Distribution Constraints
Yongli Mou, Jiahui Geng, Feng Zhou, Oya Beyan, Chunming Rong, and Stefan Decker, “pFedV: Mitigating Feature Distribution Skewness via Personalized Federated Learning with Variational Distribution Constraints,” in Lecture notes in computer science, 2023, pp. 283–294. doi: 10.1007/978-3-031-33377-4_22.
Graph Pooling Provably Improves Expressivity
Veronica Lachi, Alice Moallemy-Oureh, Andreas Roth, and Pascal Welke, “Graph pooling provably improves expressivity,” OpenReview. https://openreview.net/forum?id=lR5NYB9zrv
Mask4D: Mask Transformer for 4D Panoptic Segmentation
Julian Spravil, Sebastian Houben, Sven Behnke, “MASK4D: Mask transformer for 4D panoptic segmentation,” arXiv (Cornell University), Jan. 2023, doi: 10.48550/arxiv.2309.16133.
Towards FAIR Data in Distributed Machine Learning Systems
Yongli Mou et al., “Towards FAIR Data in Distributed Machine Learning Systems,” IEEE Global Communications Conference 2023, Dec. 2023, doi: 10.1109/globecom54140.2023.10437414.
Distilling Influences to Mitigate Prediction Churn in Graph Neural Networks
Andreas Roth and Thomas Liebig, “Distilling influences to mitigate prediction churn in graph neural networks,” arXiv (Cornell University), Jan. 2023, doi: 10.48550/arxiv.2310.00946.
Dynamic 3D Gaussians: Tracking by Persistent Dynamic View Synthesis
Julian Spravil, Sebastian Houben, Sven Behnke, “Dynamic 3D Gaussians: Tracking by persistent dynamic view synthesis,” arXiv (Cornell University), Jan. 2023, doi: 10.48550/arxiv.2308.09713.
Composing and Validating Large-Scale Datasets for Training Open Foundation Models for Audio
Marianna Nezhurina et al., “Composing and validating Large-Scale datasets for training open foundation models for audio,” journal-article. Online. Available: https://mlforaudioworkshop.com/CompValDataFoundationModels.pdf
Sustain.AI: a Recommender System to analyze Sustainability Reports
L. Hillebrand et al., “sustain.AI,” ACM Digital Library, Jun. 2023, doi: 10.1145/3594536.3595131.
Towards Linguistically Informed Multi-objective Transformer Pre-training for Natural Language Inference
Maren Pielka, Svetlana Schmidt, Lisa Pucknat, and Rafael Sifa, “Towards linguistically informed multi-objective transformer pre-training for natural language inference,” in Lecture notes in computer science, 2023, pp. 553–561. doi: 10.1007/978-3-031-28238-6_46.
Improving Natural Language Inference in Arabic Using Transformer Models and Linguistically Informed Pre-Training
Maren Pielka, Jörn Hees, Bouthaina Soulef Abdou, Rafet Sifa, and Mohammad Majd Saad Al Deen, “Improving natural language inference in Arabic using transformer models and linguistically informed Pre-Training,” IEEE Conference Publication | IEEE Xplore, Dec. 05, 2023. https://ieeexplore.ieee.org/document/10371891
Generating Prototypes for Contradiction Detection Using Large Language Models and Linguistic Rules
Maren Pielka, Svetlana Schmidt, and Rafet Sifa, “Generating Prototypes for Contradiction Detection Using Large Language Models and Linguistic Rules,” IEEE Xplore, Dec. 2023, doi: 10.1109/bigdata59044.2023.10386499.
Measurability of quality characteristics identified in latent spaces of Generative AI Models
Robert H. Schmitt, Dominik Wolfschläger, Jan-Henrik Woltersmann, and Lennart Stohrer, “Measurability of quality characteristics identified in latent spaces of Generative AI Models,” CIRP Annals, Jan. 2024, doi: 10.1016/j.cirp.2024.04.073.
Language models scale reliably with over-training and on downstream tasks
Marianna Nezhurina and Jenia Jitsev, “Language models scale reliably with over-training and on downstream tasks,” arXiv (Cornell University), Mar. 2024, doi: 10.48550/arxiv.2403.08540.
Improving Zero-Shot Text Matching for Financial Auditing with Large Language Models
Lars Hillebrand, Armin Berger, Tobias Deußer, Tim Dilmaghani, Mohamed Khaled, Bernd Kliem, Rüdiger Loitz, Maren Pielka, David Leonhard, Christian Bauckhage, and Rafel Sifa, “Improving Zero-Shot Text Matching for Financial Auditing with Large Language Models,” ACM Digital Library, Aug. 2023, doi: 10.1145/3573128.3609344
OpenFlamingo: An Open-Source Framework for Training Large Autoregressive Vision-Language Models
Jenia Jitsev et al., “OpenFlamingo: an Open-Source framework for training large autoregressive Vision-Language models,” arXiv (Cornell University), Jan. 2023, doi: 10.48550/arxiv.2308.01390.
HyenaPixel: Global Image Context with Convolutions
Julian Spravil, Sebastian Houben, and Sven Behnke, “HyenaPixel: Global Image Context with Convolutions,” arXiv (Cornell University), Feb. 2024, doi: 10.48550/arxiv.2402.19305.
Gated Temporal Diffusion for Stochastic Long-Term Dense Anticipation
Olga Zatsarynna, Emad Bahrami, Yazan A. Farha, Gianpiero Francesca, and Juergen Gall, “Gated temporal diffusion for stochastic Long-Term dense anticipation,” arXiv (Cornell University), Jul. 2024, doi: 10.48550/arxiv.2407.11954.
How Much Temporal Long-Term Context is Needed for Action Segmentation?
Emad Bahrami, Gianpiero Francesca, and Juergen Gall, “How Much Temporal Long-Term Context is Needed for Action Segmentation?,” arXiv.org, Aug. 22, 2023. https://arxiv.org/abs/2308.11358
Chronicling Germany: An Annotated Historical Newspaper Dataset
Christian Schultze, Niklas Kerkfeld, Kara Kuebart, Princilia Weber, Moritz Wolter, and Felix Selgert, “Chronicling Germany: an annotated historical newspaper dataset,” arXiv.org, Jan. 30, 2024. https://arxiv.org/abs/2401.16845
Get in touch with us!
Do you have any questions or are you interested in working with us?
Feel free to email us, and we’ll be happy to help and advise you.
Follow us on LinkedIn
Don’t want to miss WestAI updates, news, and events or want to share them faster with your network?
Follow us on LinkedIn!